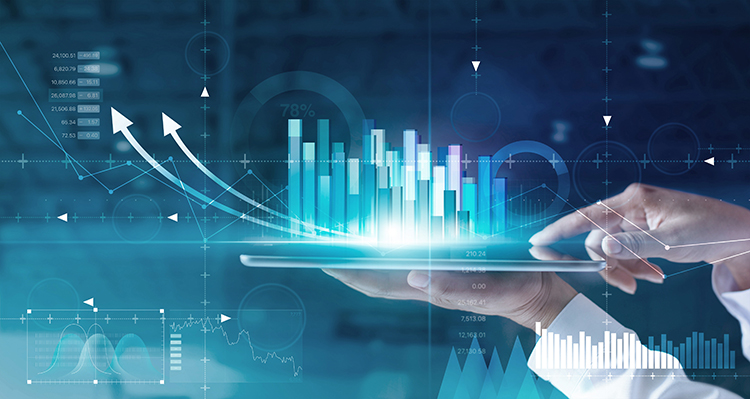
Making good decisions requires having good information. However, it also requires interpreting that information appropriately and accurately. When the wrong conclusions are drawn, we might expend resources on solving a problem that doesn’t actually exist. Worse yet, we might fail to recognize a problem and intervene to fix it.
Even in small sample sizes, it’s possible to misinterpret pieces of information, and with the abundance of data dairy farmers can now collect on their animals, there’s more to sort through when making decisions. To avoid making mistakes from interpreting your data, follow these five tips as outlined by Mike Overton of Zoetis during the Data and Innovations Summit:
1. Devise your question. What are you looking to answer from digging into the data you have available? This question should be appropriate for whatever response is happening in the herd to trigger your concern. Keep it concise — why might this be happening, how long has this been happening, what is the trend for this metric, and so on.
2. Identify the data you want. Next, determine what metrics will help answer your question. Do you want to look at information for a certain group, or a certain period of lactation? You may need to determine if you even have information about what you need to analyze. When choosing what areas to track, keep in mind the variable results of a group and don’t always assume changes are real. Especially in an area such as reproduction, randomness occurs and might make something look like a trend that’s really not.
3. Collect data. The actual collection of the data — such as milk production, disease incidence, and pregnancy rates — is probably already being recorded in some form on your farm. It is then the reviewer’s job to screen the information to be sure it is recorded accurately and without bias, or some kind of systematic error. An example of bias may be in how health conditions are entered into a herd software. If the only cows that get recorded as being ketotic are the ones that require the most attention and treatment, the problem may look more severe than it is when based solely on records. In reality, most ketotic cows may be requiring so little treatment that they are not even recognized in the system. Bias is also introduced in outliers. One cow with an extremely high somatic cell count would raise the average of the herd significantly. Without eliminating these outliers, averages won’t show a very accurate picture.
4. Analyze the data appropriately. Think of this broad step as the place to slow down. Analyzing appropriately means being aware of all the other factors influencing the numbers you’re looking at. Know the time frame represented in the data, and when comparing two sets, make sure they are on the same schedule. Consider any confounding variables that may be affecting the data you have but aren’t directly tied to the question you want to answer.
5. Interpret and form a plan. Finally, draw your conclusions from what you’re seeing, remembering that correlation does not equal causation. Other than milk production and genetic information, Overton said he is not a fan of benchmarking with other herds to interpret your data because no two farms operate or record their information exactly the same. Compare your own performance over time, and in the case of disease, work with your vet to regularly record incidences accurately. For smaller dairies with fewer data points on a certain incident, bring in information from more sources related to the overall area you’re evaluating to get a fuller picture. Uncovering what’s actually happening on the farm will help you make the most effective plan to move forward.