The collaborators and authors of this article include Michelle Axford, Victor Cabrera, Jessica Cederquist, Mark Doornink, Joao Dorea, Liliana Fadul, Jerry Guenther, Andrew Maier, Jay Mattison, Mutian Niu, and Matthijs Vonder. Project supported by USDA-NIFA-FACT grant 2019-68017-29935.
Download a PDF of this article.
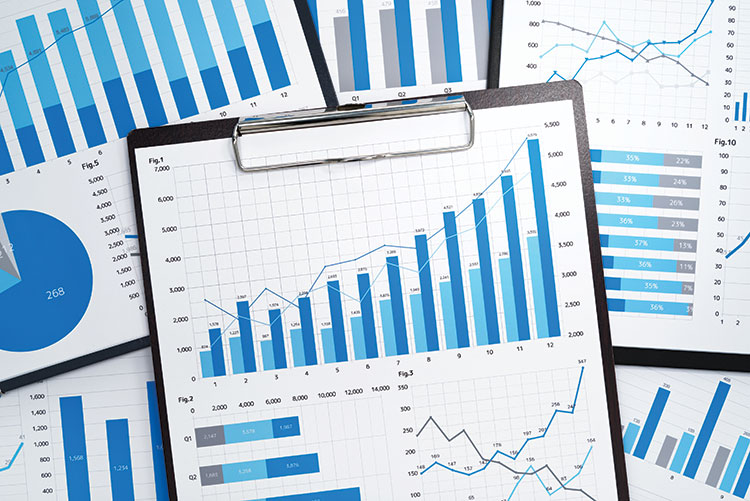
Data collection, integration, and analysis are unavoidable factors when it comes to advancing the development of decision support tools in livestock operations. Getting the most out of the data is key to help create accurate and reliable management tools at both the farm and animal level. However, in order to achieve excellence in data collection and analysis, some key points need further discussion.
As more technology becomes available, more data is generated at a trivial cost. Unfortunately, these large data streams do not arrive in an organized or integrated fashion. These aspects need to be discussed to make the most out of the data or to transform it into information . . . or even better, into working instructions or standard operating procedures that can be implemented on farm and also to make it more efficient. There are five crucial points that will be addressed in this article:
- Missing or incomplete metadata
- Data interoperability and standardization
- Types of calculation and aggregation
- Data quality (accuracy, outliers, and missing data)
- Data communication with farmers’ consent
This article is a summary of an ongoing discussion from a subgroup of the Dairy Brain’s Coordinated Innovation Network (CIN). It’s our goal to generate a larger industry discussion, and everyone is encouraged to contribute via the web portal found at the bottom of the page.
1. Missing or incomplete metadata
Metadata is data about the data. It’s important to understand the behavior of the data and extract the most relevant information of the data in both the short term and long term.
For example, the data collected over five years from one farm will potentially bring more information compared to six months of data collection. But, without metadata, in five years it will be almost impossible to accurately interpret what the variables were, what they mean, and how they were collected. In most cases, metadata is missing because the process of data collection is incomplete.
On dairy farms, one clear example of this is the lack of information about the health and culled reasons records. This being said, “information about information” is indispensable for the long-term success of today’s effort on data collection and integration.
2. Interoperability and standardization
Collected data is usually messy and almost always lacks standardization. Therefore, a protocol, preferably automated, of cleaning and harmonizing the data is one of the first steps to start working. Even though data is being generated “automatically,” many times the readings are incomplete or wrong, giving messy data as a result.
Also, lack of standardization on how variables are named is mainly caused by different software and inconsistent nomenclature to define variables. For example, a person on a farm may name mastitis as Mastitis, MST, Mast, or CMT. Furthermore, milk yields may be given in gallons, liters, or kilograms.
Such variations make it more difficult to use all the available records and harmonize the data. The greatest concern centers on data collection standards. To learn more about this topic, please refer to the first article of this series: “Help us help you make better use of dairy data” on page 82 of the February 10, 2020, issue of Hoard’s Dairyman.
3. Calculation and aggregation
Each system or software makes its own calculations and aggregations according to different periods of time or management levels such as cow, pen, or herd. That creates variables that are difficult to compare across systems. The ideal situation will be to have access to the raw data and the metadata, generated by the different software systems, to have a clear understanding of the data. And this way, the development of predictive and prescriptive analytics will be easier.
4. Data quality
In this section, there are more questions than possible answers. How is “good” data quality defined? Is the quality of the data we are getting from the different technologies good enough for the purpose that it is being used? Are there explainable outliers? Does the data arrive in time and/or is data missing? Is it affected by the maintenance of the technology and sensors? Does the technology or sensor need to be calibrated and how often? How do we tell if a sensor is failing?
Do we need to develop technologies to monitor or even improve the quality of data collection? If we do not have good quality data, then is the analysis going to be misleading? This could lead to the wrong output and subsequent wrong decision support tools. Therefore, it will be important to provide as much relevant information known as metadata about the measurements. This is a starting point to try to answer some of these questions.
5. Communication with farmers’ consent
Retrieving and aggregating data from different sources enables better decision support services. This is dependent on the willingness of the different companies to share the data with prior consent of the farmers. Establishing the benefits of data sharing to farmers and to data suppliers is the first step for making data communication happen.
Once this is agreed upon, the next step is to establish protocols to make data communication happen. Data from various systems are stored and operated individually, therefore, reliable data transfer methods need to be developed.
Certain protocols that govern the communication should be set to ensure the delivery and accuracy of data in a timely manner. Integrated data should be easily queried/accessed and shared by different users. Further, the development of application programming interfaces (APIs) is the direction to facilitate data sharing.
What does it all mean?
Putting it all together, and helping farmers make the most of their data, we should “think big but start small.” We need to be aware that there are already a lot of data types and they are evolving over time. It is clear there will be more diverse and other data types available. Hence, developing a system based on an architecture that can enhance functionality and scale up (more farmers, more farms, more animals, and more sensor types), with a minimum of manual labor to do so, will be the ideal scenario for data communication. New data streams should be stored automatically, with minimal human handling.
Share your input with the Dairy Brain team To contribute to the discussion, go to on.hoards.com/DairyBrainForum or scan the QR code with your smartphone.