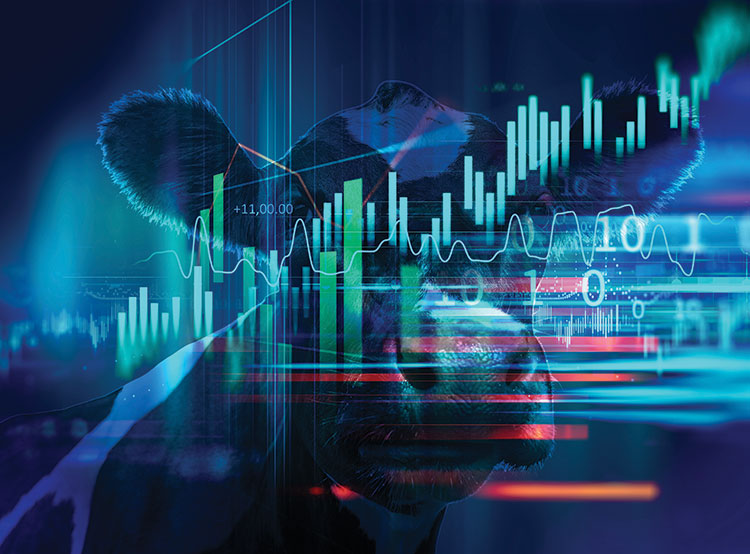
Data is a key driver for improving operations and sustainability of physical and business systems. While other articles in this series have addressed issues related to acquisition, security, and ownership of data, this article concentrates on the valuation of an exchange and enhancement of that data. In the context of dairy, who should exchange what data, why, and how should this be facilitated?
The ultimate goal with all of these activities is to create more value for farmers, dairy processors, and for all consumers. The value created should be shared with those carrying out the value-added activities, which could range from existing milking machine companies and farmer co-ops to cloud computing firms and technology start-ups. The beneficiaries of the value creation will pay those supplying the technology, products, and services that enable it — the underlying basis for a data mart.
Data and its movement
Broadly speaking, farm data is moved through a value chain, starting with acquisition on the farm (including sensorization of components), cleaning and transformation into a usable format, and storing it reliably and in ways that promote easy access. Different complementing data streams are joined together and processed via analytics to provide outputs, insights, and decision support.
Each of these transformation steps potentially add value to the data and determining where farmers and companies pay for added value that such services provide is central to this discussion. It goes without saying that communication of the results is also critical; different visualizations and summaries that are phrased in the language of the dairy industry also add value.
The figure illustrates the vision for a prototypical integrated system. It consists of an Agricultural Data Hub (AgDH), in blue, that carries out the first four tasks in the value chain. There is a collection of applications that we term Dairy Brain, shown in red, which serve to process those data streams to provide definitive outputs or insights.
A prototypical agricultural data hub
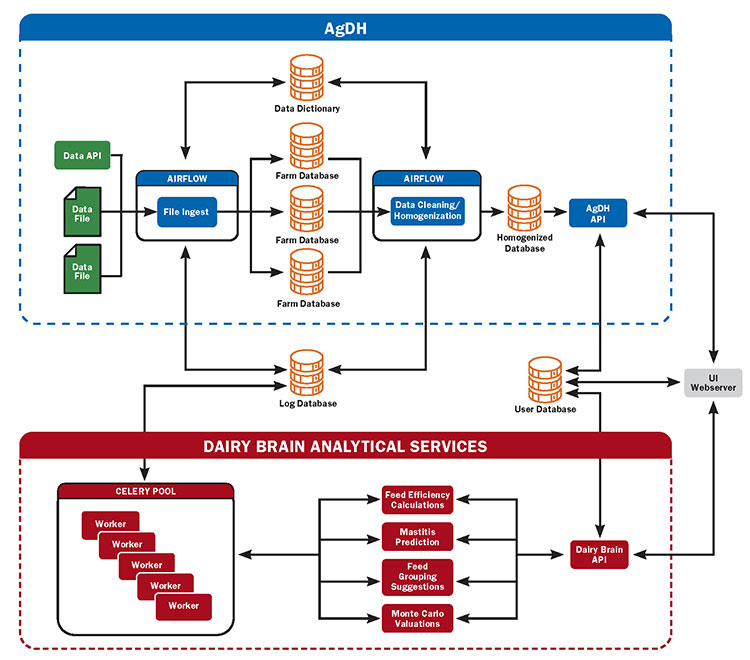
The AgDH acts as a data mart, or some market-based system to facilitate the exchange of data at various levels of refinement and provides access to data that is used to add value via analytics, aggregation, or inference. It is the exchange that monetizes the added value, generating an alignment of groups that share data up and down the value chain. How can that market operate effectively?
Modern software applications have a series of interfaces that allow them to exchange data with other software applications, termed application programming interfaces or APIs. Their spatial location is largely irrelevant. The data is exchanged in a predefined format that is interpretable by the consuming software application.
When the data is drawn from a source application and consumed by the target application, it can be married with other data in the target application to generate insights that would not be possible if it came from just one application. Additionally, all this can potentially be carried out in real-time. Open data exchange between completely disparate software systems is the value that APIs bring to the table. Markets are built on such well-defined exchanges.
Creating success
In any industry, there are a number of issues that are critical to address in order for such a system to succeed:
Coordination: How can we encourage participation and have many data providers and users interact with the system? To create a winning situation, companies could develop interdependent products and services, and users such as farmers can benefit from the synergies between their different types of data and expertise. In this scenario, developers can recoup or offset some of the costs of their investment in sensors or cameras or other data capture technologies.
Interoperability: Can we provide application programming interfaces and data management standards that reflect the agricultural domain characteristics but are also relatively inexpensive to instantiate?
Demonstration: Are there concrete examples that show what is possible with such data streams? This then opens the opportunity for new entrepreneurs that are potentially linked with individual farms or groups of farmers to develop new software tools or other services based on their creative ideas or specific needs.
Efficiency: Are the transformations that are carried out, both during the curation and the analytics phases, performed in a timely, cost-effective, and reliable fashion? Otherwise their value becomes negligible.
Monetization: APIs are like gates — they could be free or they could be tolled — ultimately, it comes down to what value they create; for who and based on what metric.
A pathway forward
The University of Wisconsin-Madison Dairy Brain’s project is building the system outlined in the figure. The university is independent of any one vendor; it has a long history of expertise in the dairy industry, and it has institutional support to make this happen. The AgDH plus Dairy Brain is a sandbox for implementation of the actual system, and it is flexibly designed to allow many new components (both data producers and consumers) to be engaged. Along with serving as an example, there is a drive to make this project sustainable and extensible via documentation and compartmentalized implementation.
So, how can such a system and the wider availability of data exchange through APIs play a role in the dairy industry? The dairy industry already has an ecosystem of partners that create a value chain as the milk moves from entity to entity, from farm to retail. Each member along that value chain is a potential source of infinite data that could be harnessed to generate valuable insights at every level. Three examples of how the use of APIs can add value at different parts of the value chain are as follows:
The net present value (NPV) of a cow is a strategic long-term decision support tool (DST) to assess the cow’s long-term value. The NPV will help the farmer to evaluate management decisions such as breeding, determining a treatment decision if the cow becomes sick, or deciding to remove the cow from the herd. However, to calculate this value, data from five different data sources is needed (management, milking parlor, feed, milk processor, and economics). To calculate this value for all cows on a continuous basis is time consuming. The data connections and integrations of the AgDH and the Dairy Brain generate an NPV value of each cow that a farmer can use to make a strategic decision.
Product differentiation can be provided, for example, by a large retailer wishing to offer a premium milk product that includes supply provenance information. The retailer could request this information directly from the AgDH via an API call, rather than through a complicated search process.
Clinical mastitis (CM) is the most common and costly disease in dairy cows. The aggregation of milking parlor and management software data by the AgDH provides the ability to implement a machine-learning model to predict the onset of CM. The model will generate an alert with a list of cows at risk of contracting CM, at which time the farmer can better decide the subsequent course of action.
Start small, go big
These are a small sample of examples to illustrate the potential value of interconnecting new data streams. While an implementation of the system permits the more fluid transfer of data, it brings to the forefront questions about data security and ownership, value propositions, monetizing data curation and processing, and suggests a market mechanism to encourage innovation over the data value chain. Addressing these issues is critical to ensure the adoption of such a system, and the Coordinated Innovation Network (CIN) of the Dairy Brain project aims to build consensus across all interested parties.
Share your input with the Dairy Brain team
To contribute to the discussion, go to on.hoards.com/DairyBrainForum.