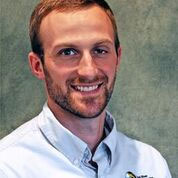
With dirty sunglasses, your field of view is often clouded and limited. If you’re anything like me, dirty sunglasses are a pet peeve that you can’t bear for much more than a few minutes before cleaning. In fact, when I pick up my wife’s dirty sunglasses it drives me nuts! We’ll leave that discussion for another day.
Data can be dirty, too
In both cases above, some amount of dirtiness can make our lives more difficult. The first case ties into lost efficiency and performance, and the second ties into vision. In recent board meeting discussions with a herd management software team, I’ve learned that data can also be dirty. This didn’t immediately make sense. However, as I grasped the concept, I recognized that the ramifications associated with dirty data are much like that described above with fuel or glasses. Dirty data clouds our ability to see what’s going on and can contribute toward efficiency challenges.
Moving to the positive, the power associated with clean data is immense. Clean data will yield a clear vision of what is really happening on your farm, or with field trials you conduct. As I’ve come to understand, clean data is well defined within a central database. In layman’s terms, this means that whatever the data item is called is clear and consistent, and in one spot. This can be in the cloud.
Many of the software programs we use are based in the cloud, or making a move to the cloud. The cloud is a central spot where data and information can be stored.
What’s the cloud?
Think about this as having 10 different toolboxes laying around your farm, and making an effort to clean up and organize all of your tools in one central toolbox. With one central toolbox, everything is organized together and in the right place.
Cloud-managed data is very similar: It is organized and defined with a single spot for software programs to work with. This central and clean data is powerful and unlocks the ability to quickly extract insight from the data.
These concepts become relevant for your farm when we get into benchmarking, evaluating protocols, or making comparisons to decide which approach was more profitable. This can be within your farm or when comparing your farm to others. We do this all the time and use powerful computer software programs to unlock the power held in your data, to streamline the path to the right decisions for your farm.
Your data evaluations could be tied into cow level data like fresh cow events, or pen level data like milk production. You and your advisers may also evaluate feeding and nutrition data or make hybrid comparisons. In any of these cases, comparing apples to apples is important.
Challenges arise when the data is dirty. If one pen or farm labels dry matter intake “DMI” but another pen or farm labels this parameter “intake,” then pulling these two data sets into a larger comparative data table is more difficult. The data analyst needs to decode the data column headings prior to comparing the two farms.
In another example, if one farm labels their brown midrib silage “BMR” but another with brown midrib silage labels it “Bunker 3,” then we can’t make the comparison at all. We don’t know what “Bunker 3” really is when searching the database. This silage description challenge is all too common. Many have asked what hybrids or varieties perform well nutritionally, but commercial forage databases are smudged with ill-defined sample descriptions.
On a higher level, this dirty data realization has played out numerous times lately, as I’ve been asked to help compare hybrids, evaluate total mixed rations from different farms relative to milk components, and compare different dairy farms’ forage quality in benchmarking. In all of these cases, data descriptions weren’t clear and are examples of dirty data. The needed data was tough to match up. Unfortunately, there are many, many examples like these throughout agriculture, from the soil to the bulk tank. However, our industry is making strides by improving data integrity.
Multiple tie-ins
This topic is complex but ties into every aspect of your farm. Take some time and think about how you define meaningful events and protocols or label samples. Ask your advisers for their thoughts and talk about your options to centralize your data.
As we continue into the future with a margin and business-minded focus, well-defined and centralized or cloud-held data will help us identify margin and efficiency opportunities. With cleaner data, we can more effectively identify the best performing dairy site, hybrid, treatment, nutrition or management approach, fresh cow or reproductive protocol, or feeder.
There may be many other evaluations on your farm, but determining the winning strategy will certainly be streamlined with a clean data analysis. Your farm profitability is fueled by crunching numbers much like a tractor engine is fueled by diesel. Keeping both clean will improve your farm’s long-term performance.